Exploring Affective Computing Concepts
Introduction
Emotional computing isn’t a new field of research. For decades computer scientists have worked on modelling, measuring and actuating human emotion. The goal of doing this accurately and predictively has so far been elusive.
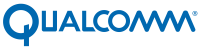
In the past years we have worked with the company Qualcomm to create intellectual property related to this topic, in the context of health care and the automotive space. Even though this project is pretty off-topic from our ususal focus areas it is an interesting sidetrack that I think is worth posting about.
Affective Computing Concepts
As part of the program we have worked on various ideas ranging from relatively simple sensory devices to complete affective control systems to control the emotional state of a user. Two examples of these approaches to emotional computing are shown below.
The Skin Color Sensor measures the color of the facial complexion of a user, with the goal of estimating aspects of the emotional state of the person from this data. The sensor is to have the shape of a small, unobtrusive patch to be attached to a spot on the forehead of the user.
Another affective computing concept we have worked on is the Affectactic Engine. A little device that measures the emotional state of a user via an electromyography sensor and accelerometer. Simply speaking we are imagining that high muscle tension and certain motion patterns correspond to a stressed emotional state of the user or represent a “twitch” a user might have.
The user is to be reminded of entering this “stressed” emotional state by vibrations emitted from the device. The device is to be attached to the body of the user by a wrist band, with the goal of reminding the user of certain subconscious stress states.
Patents
In the course of this collaboration we created several groundbreaking patents in the area of affective computing: